Image Annotation for Machine Learning: Unlocking Potential in AI
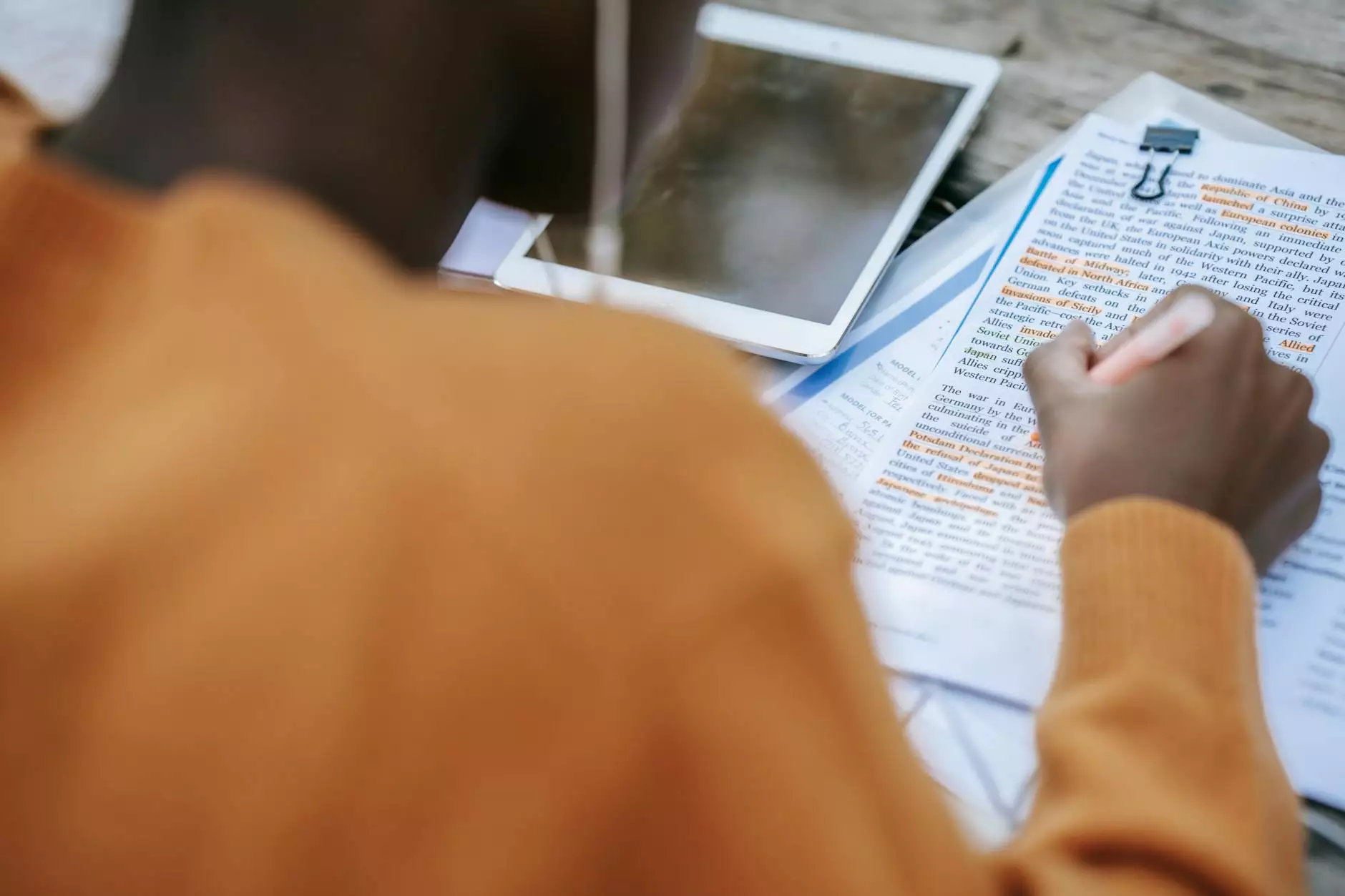
In the realm of machine learning, images play a pivotal role. As AI systems become increasingly capable, the need for high-quality training data has never been more crucial. Among various data types, images serve as a fundamental input for many applications, from object recognition to facial recognition. To leverage these images effectively, one must engage in image annotation for machine learning.
Understanding Image Annotation
Image annotation is the process of labeling images to help machine learning models understand and learn from the data. This step is essential, as it translates visual data into a format that algorithms can interpret. Different annotation techniques suit different applications, including:
- Bounding Boxes: Drawn around objects to define their position.
- Semantic Segmentation: Divides an image into segments and labels each segment.
- Keypoint Annotation: Marks specific points on objects, useful for pose estimation.
- Polygons: For irregularly shaped objects that cannot be encompassed by bounding boxes.
The Role of Image Annotation Tools
The efficiency of image annotation for machine learning is greatly influenced by the tools available. Here, we explore various tools that facilitate the annotation process:
1. Keylabs.ai Annotation Tool
Keylabs.ai offers a powerful platform for image annotation tailored for machine learning projects. With features designed for precision and ease of use, this tool empowers annotators to create accurate datasets efficiently. Key features include:
- Intuitive Interface: A user-friendly interface that simplifies the annotation process.
- Collaboration Features: Teams can work together seamlessly, enhancing productivity.
- Real-time Feedback: Immediate visual feedback helps improve accuracy during the annotation process.
- Automation Options: Integrates advanced algorithms to automate repetitive tasks, saving time and resources.
2. Open-source vs. Proprietary Tools
When choosing an image annotation tool, one must consider the benefits of both open-source and proprietary solutions. Open-source tools promote community collaboration and flexibility, while proprietary tools, like Keylabs.ai, often offer superior support and advanced features. Here’s a comparative analysis:
FeatureOpen-source ToolsProprietary Tools (Keylabs.ai)CostFreeSubscription-basedSupportCommunity-basedDedicated support teamsCustomizationHighly customizableLimited customizationFeaturesBasic featuresAdvanced featuresWhy is Image Annotation Important for Machine Learning?
The significance of image annotation for machine learning cannot be overstated. Here are several reasons why it is a vital component:
1. Enhances Model Performance
By providing accurate and meaningful labels, image annotation directly contributes to the performance of machine learning models. Models trained on well-annotated data demonstrate higher accuracy and reliability.
2. Supports Diverse Applications
Image annotation enables a variety of applications across numerous domains, including:
- Autonomous driving systems (object detection).
- Healthcare applications (identifying tumors in medical images).
- Retail (customer behavior analysis through image recognition).
- Social media (image tagging and categorization).
3. Aids in Legal and Compliance Obligations
With increasing regulations around data usage, proper image annotation practices help organizations maintain compliance, ensuring that data usage aligns with legal standards.
Best Practices for Successful Image Annotation
1. Define Clear Guidelines
Establishing comprehensive guidelines for annotators ensures consistency and accuracy in the labeling process. Clear examples of desired outputs can significantly improve the quality of annotations.
2. Utilize Multiple Annotators
Engaging multiple annotators can help identify discrepancies and improve the overall quality of annotations. Consensus from various annotators can lead to a more robust dataset.
3. Implement Quality Control Measures
Regularly reviewing and validating annotations helps maintain high-quality standards. Implementing feedback loops where annotators receive critiques can further enhance their performance.
4. Leverage Technology
Incorporating AI and machine learning algorithms into the annotation process can streamline workflows, automate repetitive tasks, and improve efficiency. For instance, semi-automated annotation tools can significantly reduce the time spent on repetitive labeling tasks.
Choosing the Right Data Annotation Platform
When selecting a data annotation platform, it’s crucial to assess your specific needs and goals. Keylabs.ai presents an optimal solution for organizations looking for efficient and scalable image annotation for machine learning. Here are some factors to consider:
1. Scalability
The chosen platform should accommodate varying project sizes, from small datasets for proof-of-concept to large-scale annotations needed for extensive machine learning applications.
2. Flexibility
Different projects may require different types of image annotation. Choose a platform that can handle various annotation styles and formats.
3. Integration Capabilities
Ensure that the platform can integrate with your existing workflows and tools. This will make the transition smoother and allow for a better overall experience.
Future Trends in Image Annotation for Machine Learning
As technology continues to evolve, so too does the field of image annotation. Here are some emerging trends that are shaping the future of this pivotal aspect of machine learning:
1. AI-Assisted Annotation
Machine learning algorithms that assist in the annotation process are becoming increasingly common. These systems can speed up the initial labeling and allow human annotators to focus on refining the data.
2. Crowdsourced Annotation
Crowdsourcing approaches to image annotation are gaining traction, enabling faster processing times while allowing for diverse input from a global pool of annotators.
3. Virtual and Augmented Reality Annotations
As VR and AR technologies mature, their applications in image annotation are also expanding, providing new ways to visualize and label data in a more interactive manner.
Conclusion: The Path Forward for Image Annotation in Machine Learning
In summary, image annotation for machine learning is not merely a technical requirement; it is a foundational necessity that directly impacts the success of AI projects. The combination of effective tools, clear guidelines, and quality control measures sets the stage for outstanding results. By utilizing platforms like Keylabs.ai, businesses can not only enhance their machine learning efforts but also drive innovation across various domains.
As the demand for high-quality annotated data grows, organizations must prioritize effective strategies for annotation to stay competitive. Embrace the future, invest in the proper data annotation tools, and watch as your machine learning projects flourish.